CS2750: Machine Learning (Spring 2025)
- Class time: Monday & Wednesday 11:00am – 12:15pm
- Class location: A215 Public Health-Crabtree
- Instructor: Ryan Shi
- Email: ryanshi@pitt.edu
- Office location: Sennott Square 5415
- Office hours: Wednesday 4 - 5pm and by appointment
- TA: Maneesh Bilalpur, mab623@pitt.edu
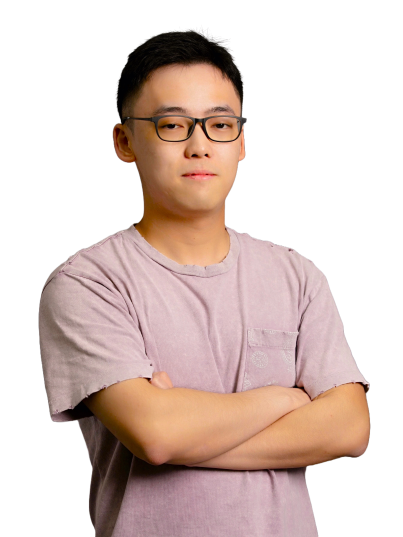
Course Description
This PhD-level course on machine learning provides an in-depth exploration of theoretical foundations, algorithmic innovations, and practical applications of machine learning. The course emphasizes mathematical rigor, algorithmic efficiency, and the ability to critically evaluate research in the field. Machine learning as a subject has evolved dramatically over the past decades. Topics covered by this class include supervised learning, deep learning, learning theory, reinforcement learning, emerging paradigms like generative AI and causal inference, and their applications to real-world social impact problems.
Prerequisites
Formal prerequisites include linear algebra, probability, algorithms, and proficiency in at least one programming language. A high level of mathematical maturity will be essential. A prior course on machine learning or AI is not required. Please see the instructor if you are unsure whether your background is suitable for the course.
Textbooks
This course does not exactly follow any one textbook. However, most lectures will have some optional reading to help you better understand the material or see a different presentation/perspective. Most of the time, these materials will be a chapter or two from the following textbooks. In other cases, we are discussing new topics that have not been written into any mature textbooks yet. We will assign research papers as we go.
- Machine Learning; Tom Mitchell.
- Dive into Deep Learning; Aston Zhang, Zack C. Lipton, Mu Li, Alex J. Smola.
Course Schedule (Subject to Change)
Paper Presentations
You will be asked to present 1 paper in class throughout the semester. The length of each presentation is expected to be around 20 minutes.
You will want to go into the technical details and make sure your classmates understand the ins and outs of the paper. You will also want to critically examine the paper and talk about its strengths and weaknesses. It might help to situate this paper in the literature by finding and reporting on at least one older paper that substantially influenced the current paper and at least one newer paper that is influenced by the current paper. It would also be helpful to propose a couple of follow-up directions that were not mentioned by the paper’s authors.
You are required to meet with the instructor to discuss the paper and the presentation, no later than 3 days before your presentation. You may use the designated office hour or email the instructor for additional times.
Course Project
You will work on a course project related to machine learning. The progress of projects will be checked through the Project Proposal, Project Progress Report, Project Presentation, and Final Project Report. The proposal and progress reports will be peer-reviewed and graded by the instructor. The presentation and the final report will be evaluated by the instructor directly.
You will work in groups of 1-2 people (TBC – depending on the class size) on an original research project on machine learning. The students are expected to focus on a particular research problem that fits in the broad set of topics covered in this course and develop novel research contributions. The final project report will get a full score if at the level acceptable to a competitive workshop at a major AI conference. You are encouraged to align the course project with your own research agenda.
Proposal due: February 7th
Progress report due: March 21st
Oral presentations: April 21st
Final report due: April 28th
Grading
Course Component | Percentage of Final Grade |
---|---|
Class participation | 10% |
Paper presentations | 10% |
Quiz | 20% |
Project proposal | 10% |
Project progress report | 10% |
Project oral presentation | 10% |
Project final report | 30% |
Course Policies
Grading
- Late-submission policy: You have a total of 3 late days for course assignments. Late days cannot be used towards the project-related assignments. The instructor reserves the right not to grade late submissions beyond the allowed late days.
- Re-grading policy: To request a re-grade, please write an email to the instructor titled “Re-grade request from [Student's Full Name]” within one week of receiving the graded assignment.
Collaboration
- For the course project, you may collaborate with others outside the class (including students, faculty members, and domain experts) with approval from the instructor. You are still expected to take the leading role in the project. If you have external collaborators, you need to give proper credits to all parties involved, and report the contributions of each contributor in the proposal, progress report, final report, and presentations, which will be considered in the grading.
Academic Integrity
- For the paper presentations, it is allowed to borrow presentation materials from public sources so long as the original source is acknowledged.
- For the course project, it is allowed to use publicly available code packages so long as the source of the code package is acknowledged.
- All writings in the assignments should come from you and do not submit content generated by GPT or similar language models. When in doubt about what you can or cannot use, ask the instructor.
- Plagiarism in any submitted assignments is strictly prohibited. There are serious consequences. Please consult the University Guidelines on Academic Integrity.
Accommodations for Students with Disabilities
If you have a disability for which you are or may be requesting an accommodation, you are encouraged to contact both your instructor and Disability Resources and Services (DRS), 140 William Pitt Union, (412) 648-7890, drsrecep@pitt.edu, (412) 228-5347 for P3 ASL users, as early as possible in the term. DRS will verify your disability and determine reasonable accommodations for this course.
Accommodations for Students with Medical Conditions
If you have a medical condition which will prevent you from doing a certain assignment, you must inform the instructor of this before the deadline. You must then submit documentation of your condition within a week of the assignment deadline.
Statement on Student Wellness
As a student, you may experience a range of challenges that can interfere with learning, such as strained relationships, increased anxiety, substance use, feeling down, difficulty concentrating and/or lack of motivation. These mental health concerns or stressful events may diminish your academic performance and/or reduce your ability to participate in daily activities. Pitt services are available, and participation in services does work. You can learn more about confidential mental health services available on campus here. Support is always available (24/7) from University Counseling Center: 412-648-7930.